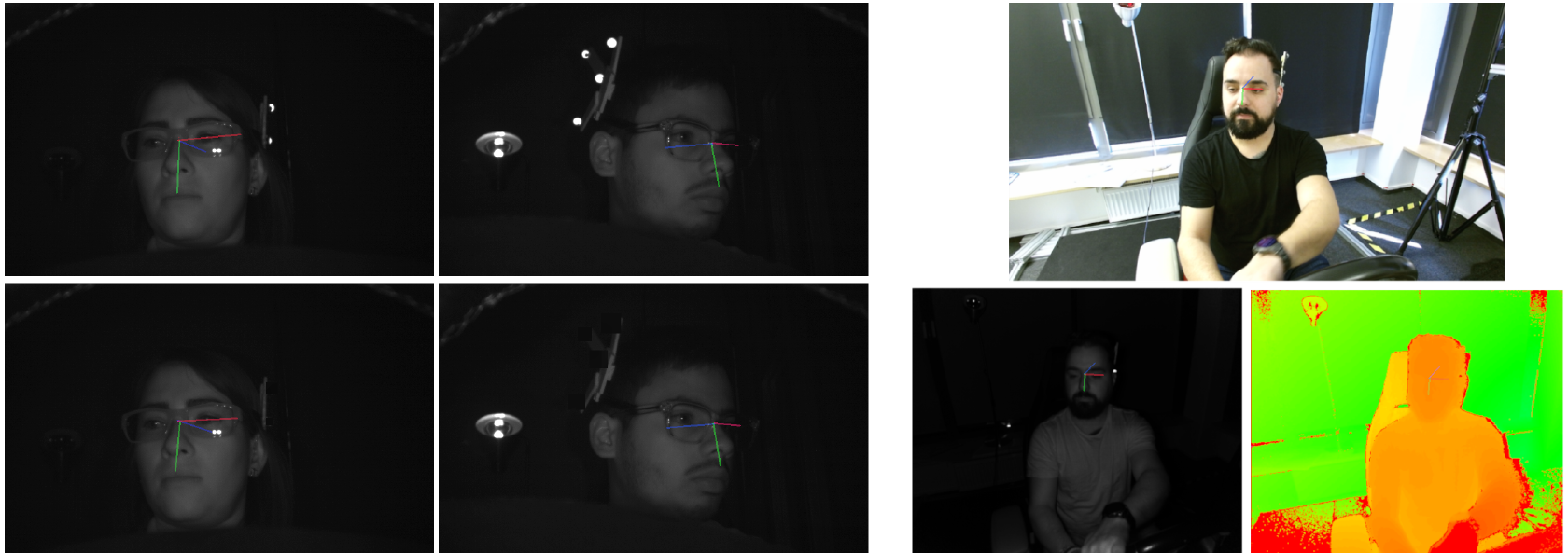
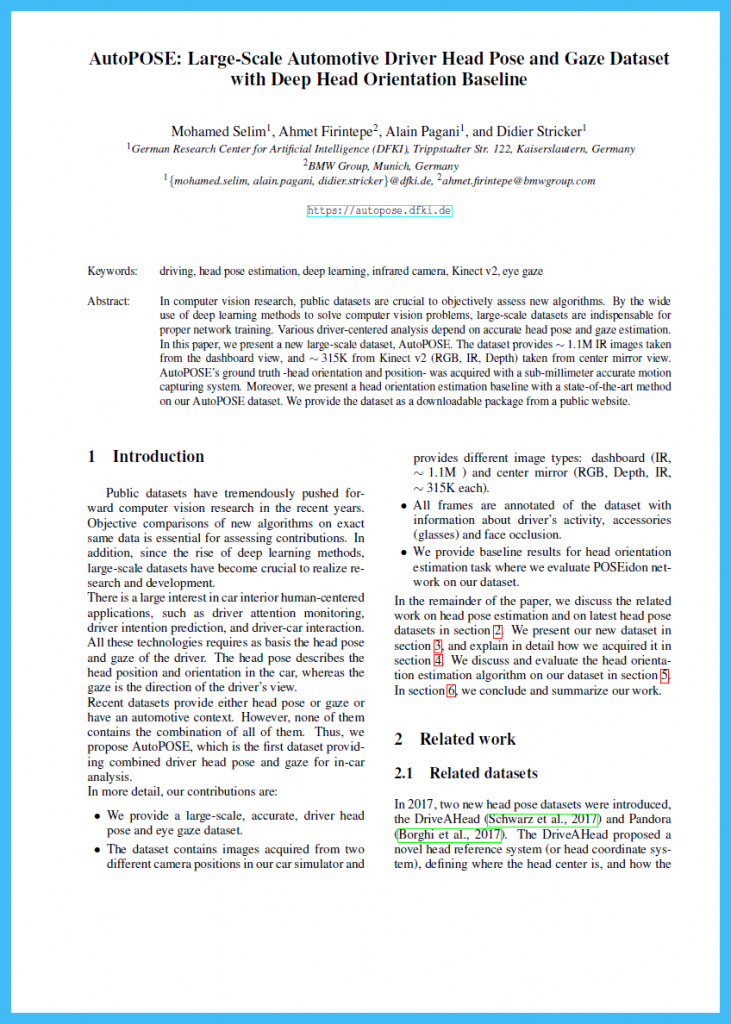
AutoPOSE dataset is published at VISAPP 2020 in the paper titled
AutoPOSE: Large-Scale Automotive Driver Head Pose and Gaze Dataset
with Deep Head Orientation Baseline
Mohamed Selim, Ahmet Firintepe, Alain Pagani, Didier Stricker
Abstract
In computer vision research, public datasets are crucial to objectively assess new algorithms. By the wide use of deep learning methods to solve computer vision problems, large-scale datasets are indispensable for proper network training. Various driver-centered analysis depend on accurate head pose and gaze estimation. In this paper, we present a new large-scale dataset, AutoPOSE. The dataset provides ∼ 1.1M IR images taken from the dashboard view, and ∼ 315K from Kinect v2 (RGB, IR, Depth) taken from center mirror view. AutoPOSE’s ground truth -head orientation and position-was acquired with a sub-millimeter accurate motion capturing system. Moreover, we present a head orientation estimation baseline with a state-of-the-art method on our AutoPOSE dataset. We provide the dataset as a downloadable package from a public website.
When using this dataset in your research, please cite us:
@INPROCEEDINGS{Selim2020AutoPOSE,
author = {Mohamed Selim and Ahmet Firintepe and Alain Pagani and Didier Stricker},
title = {AutoPOSE: Large-Scale Automotive Driver Head Pose and Gaze Dataset
with Deep Head Orientation Baseline},
booktitle = {International Conference on Computer Vision Theory and Applications (VISAPP)},
year = {2020},
url = {http://autopose.dfki.de}
}
Acknowledgement
This work was partially funded by the company IEE S.A. in Luxembourg. The authors would like to thank Bruno Mirbach, Frederic Grandidier and Frederic Garcia for their support. This work was partially funded by the German BMBF project VIDETE under grant agreement number (01jW18002).